News Story
New Wearable Sensor Modules with Edge Machine Learning for Avatar Reconstruction
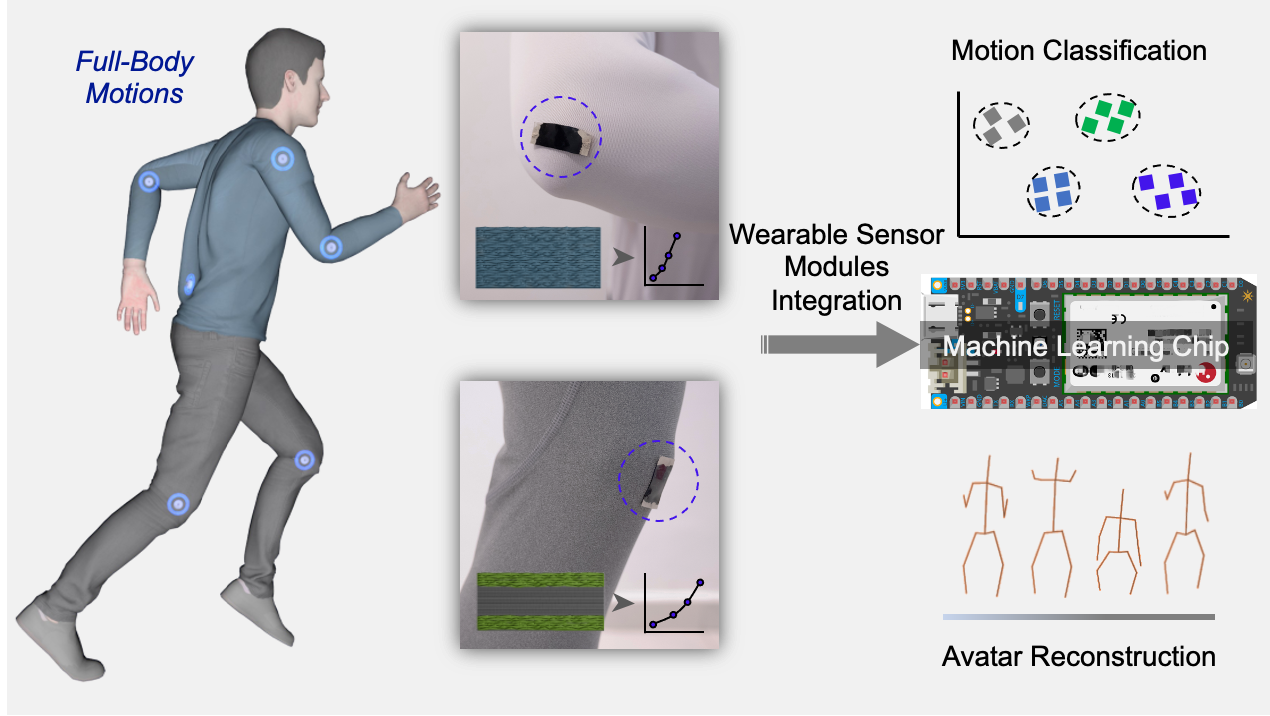
Po-Yen Chen, Chemical and Biomolecular Engineering & Maryland Robotics Center,and Assistant Professor Sahil Shah, Electrical and Computer Engineering, have published their paper in Nature Communications Journal, entitled “Topographic Design in Wearable MXene Sensors with In-Sensor Machine Learning for Full-Body Avatar Reconstruction." Nature Communications is an open access, multidisciplinary journal dedicated to publishing high-quality research in all areas of biological, health, physical, chemical and Earth Sciences.
For this paper, Shah and Chen studied wearable strain sensors that detect joint/muscle strain changes and human-machine interfaces for full-body motion monitoring. They discovered that the state-of-the-art strain sensors and commercial sensors are not able to customize sensor characteristics with the specific deformation ranges of joints and muscles, resulting in an inability to achieve optimal results. In this work, the team adopted emerging Ti3C2Tx MXene nanosheets and conducted multiple topographic designs to fabricate an ultrasensitive wearable strain sensor that captures the deformations of various human body joints.
Furthermore, with the industrial collaboration with Realtek, they integrated the wearable sensors with a wearable machine learning chip to enable in-sensor model prediction and high-precision avatar animations that mimic continuous full-body motions. The image shows the difference between camera-recorded videos vs. the avatar reconstructed using the wearable sensor module.
Such wearable systems have a wide range of applications, including athlete performance analysis, rehabilitation assessment, human-machine interaction, and personalized avatar reconstruction for augmented/virtual reality systems.
The full article can be read at this link: https://www.nature.com/articles/s41467-022-33021-5
Published September 14, 2022